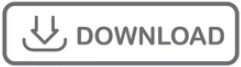
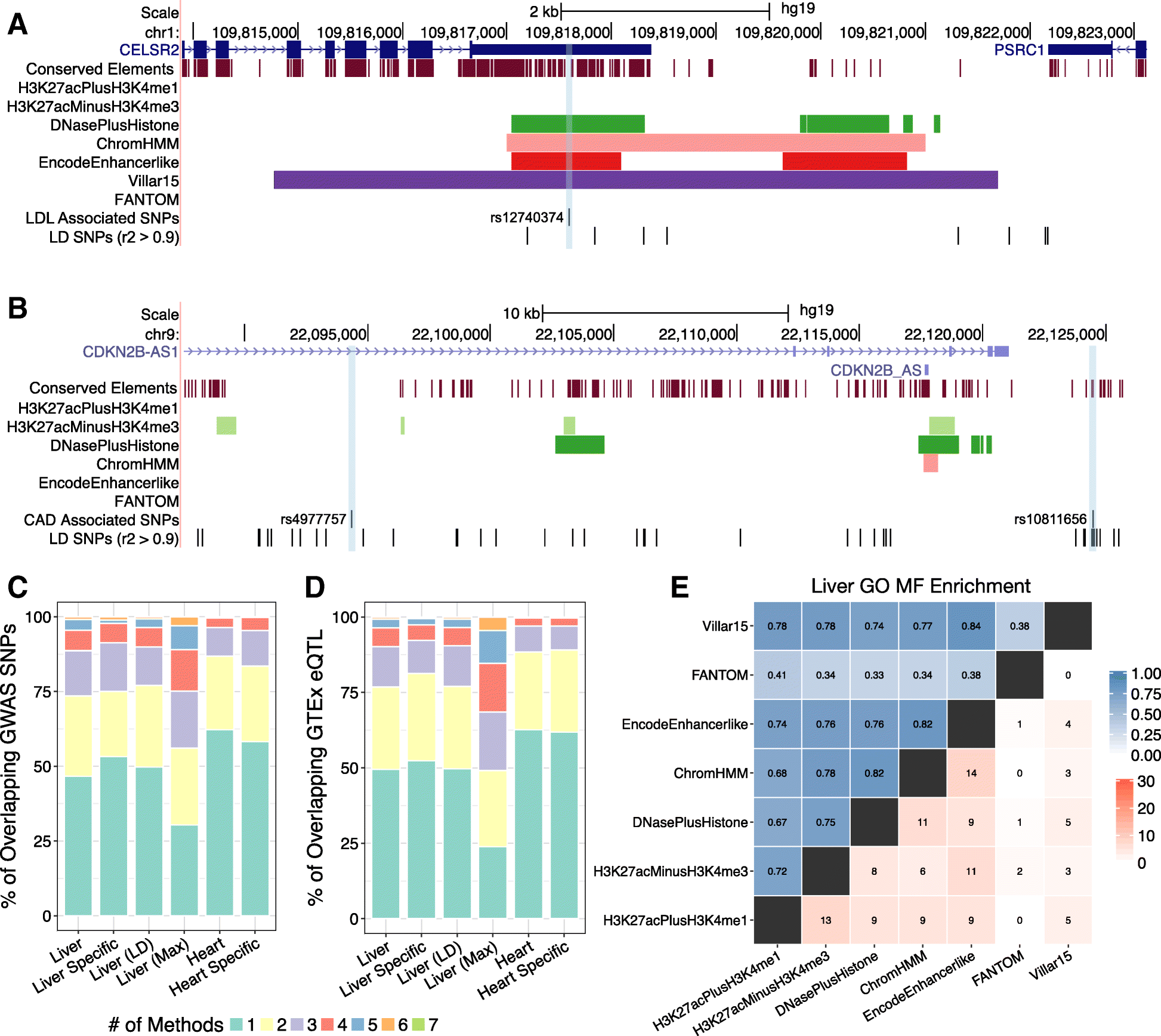
Then, multiple decoders further process this representation, each responsible for predicting a different formant while considering the lower formant predictions. Our proposed model is composed of a shared encoder that gets as input a spectrogram and outputs a domain-invariant representation. The contribution of this paper is to propose a new network architecture that performs well on a variety of different speaker and speech domains. However, when presented with a speech from a different domain than that in which they have been trained on, these methods exhibit a decline in performance, limiting their usage as generic tools. Recent work has been shown that those frequencies can accurately be estimated using deep learning techniques. Compared to the popular Wavesurfer, for example, the proposed tracker gave a reduction of 29%, 48% and 35% in the estimation error for the lowest three formants, respectively.įormants are the spectral maxima that result from acoustic resonances of the human vocal tract, and their accurate estimation is among the most fundamental speech processing problems. Results show that the proposed DNN-based tracker performed better both in detection rate and estimation error for the lowest three formants compared to reference formant trackers.
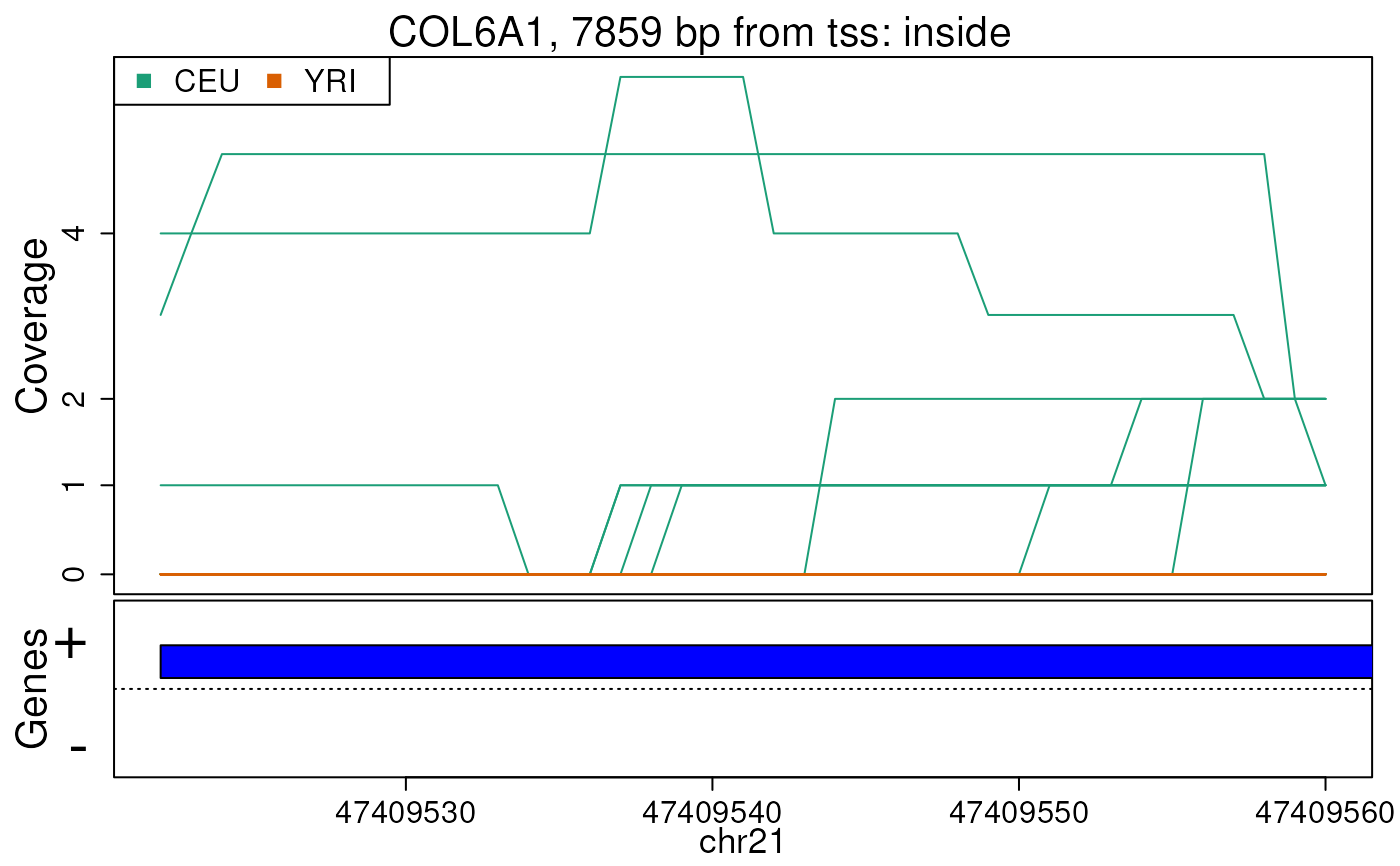
In this approach, the formants predicted by a DNN-based tracker from a speech frame are refined using the peaks of the all-pole spectrum computed by QCP-FB from the same frame. Therefore, a novel formant tracking approach, which combines benefits of deep learning and signal processing based on QCP-FB, was proposed. QCP-FB gave the best performance in the comparison. The six methods include linear prediction (LP) algorithms, weighted LP algorithms and the recently developed quasi-closed phase forward-backward (QCP-FB) method. Using the DP approach, six formant estimation methods were first compared. Formant tracking is investigated in this study by using trackers based on dynamic programming (DP) and deep neural nets (DNNs).
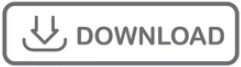